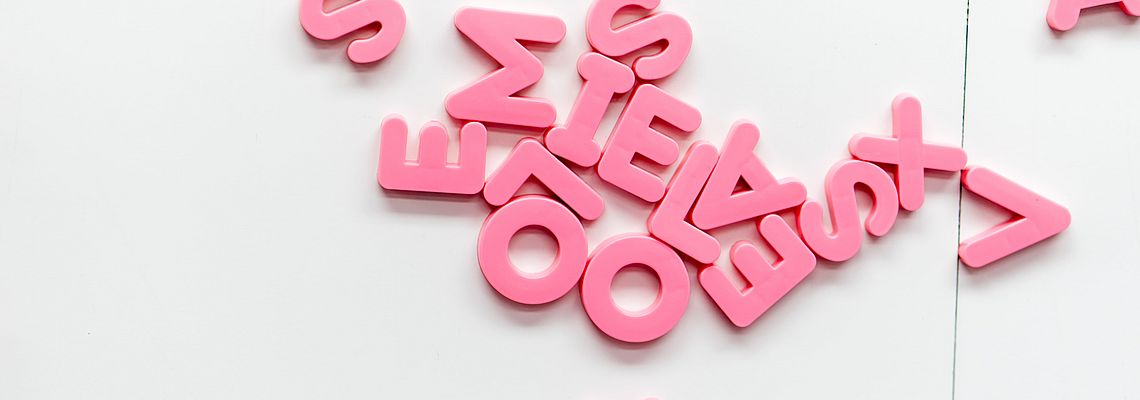
Shaping Data Into Text: How textengine.io Creates Content
Natural Language Generation (NLG) revolutionises the creation of content in digital format. In less than a second data can be turned into written text. Based on Artificial Intelligence (AI), NLG, which is also known as automated text generation, is used to create huge amounts of content in different contexts efficiently – product descriptions, weather reports, sports news or real estate reports, to name just a few. After having discussed the potentials of NLG in our last post already, let’s focus on practical matters this time: How to bring Natural Language Generation to life?
How to successfully implement Natural Language Generation?
Option 1:Leave it to a service provider like Retresco. With textengine pro, Retresco’s team of developers and linguists work with clients to outline their needs and objectives, and then build from scratch a robust complex solution that is attuned to their needs and responsive to their data. After development the solution is seamlessly integrated into an organisation’s infrastructure yet is still flexible enough for further development, improvement, and fine-tuning.
But, there is a second option, which might sound even more tempting:
Option 2: Generate your own texts with textengine.io, Retresco’s self-service Natural Language Generation (NLG) platform. Its adaptability and power gives companies and organisations the ability and resources to develop and put into place their own NLG solutions, while being supported at every step of the way. What makes this even better: textengine.io will soon be integrated in the enterprise open source TYPO3 CMS.
How does text generation with textengine.io work?
To get started with generating texts, one needs a lot of semantic data. And not just data, but structured data. Explaining what structured data is and what it means quickly leads to its counterpart, which is unstructured data. Presumably 85-90 % of all data that is available online is unstructured - text documents, audio files, videos, and images. Such content contains a range of relevant data such as personal names, locations, or quantities, but in a ‘free’ unspecified form.
Online shops, news portals, weather services, and sports sites process tremendous amounts of unstructured information. As only structured data can be managed and used efficiently for electronic data processing solutions and Internet applications, the challenge lies within managing unstructured data into further forms. Only if the data is presented in tabular forms, i.e. in columns and rows, the applications are able to use it efficiently.
A weather project, for example, relies on masses of complicated data including temperature, humidity, time, air pressure, precipitation, cloud cover, time of day, and duration. Those things all work in combination, and any change in one necessitates a whole new set of text, plus its many variations. The same goes for the eCommerce where data such as brand, design, size, price, and functionality has to be put into context to create compelling product descriptions as well as it has to be updated regularly for better visibility. While any robust system designed to do such work can fire out hundreds of texts per second, it is important from the outset that they are set up with the knowledge of how to interpret this data correctly.
For that reason, human editors need to set up the framework for text generation beforehand. To do this, they determine the sequence of the individual data information in advance in the form of prioritizations and makes an initial formatting in which they define paragraphs at relevant points. For each paragraph, they need to create templates for the wording and define conditions under which the given templates are used. Once they are in place alongside the necessary data, the system can work independently and the software engine knows how to arrange the content in a specific order, known as the ‘story plot’ or ‘narrative.’
Based on this structure many different text variants can be created, which always contain new expressions with the same statement. This can be of great importance e.g. for SEO-relevant product descriptions. Once the structure has been defined, the possibilities for variation are incredibly diverse: textengine.io enables you to generate and share report templates or product descriptions quickly, at scale, and in a personalised way. Writing content templates that reflect your brand’s tone of voice and identity, even across several languages, will encourage a continued customer engagement. You’ll attract and convert more visitors with unique, consistent, and engaging content for every product or service line.
How does textengine.io help the content creation?
Structured data is the basis for creating new content through text automation. Natural Language Generation (NLG) applications like textengine.io make it possible to import large amounts of data into a system via direct upload or API. textengine.io then allows to build and manage own NLG content. It is based on the rtr textengine, one of the world’s most-powerful pieces of software in this class and which is built to generate text in German, English, French, Dutch, and Italian. Its features include:
An intuitive interface that makes creating your own NLG projects straightforward and quick.
Automatic text adaptation through intelligent linguistic analysis takes the data provided and adjusts your text automatically.
Adaptable and easily-changeable project management, meaning that you can continually hone your NLG work, maintaining control at every step of the process.
Comprehensive onboarding and support from Retrescos customer success management to guarantee a successful project.